The healthcare industry is undergoing a period of rapid transformation, driven by advancements in technology, evolving patient expectations, and an increasing focus on personalized medicine. While significant progress has been made in improving health outcomes and extending lifespans, several challenges remain that hinder the delivery of high-quality, accessible, and affordable healthcare.
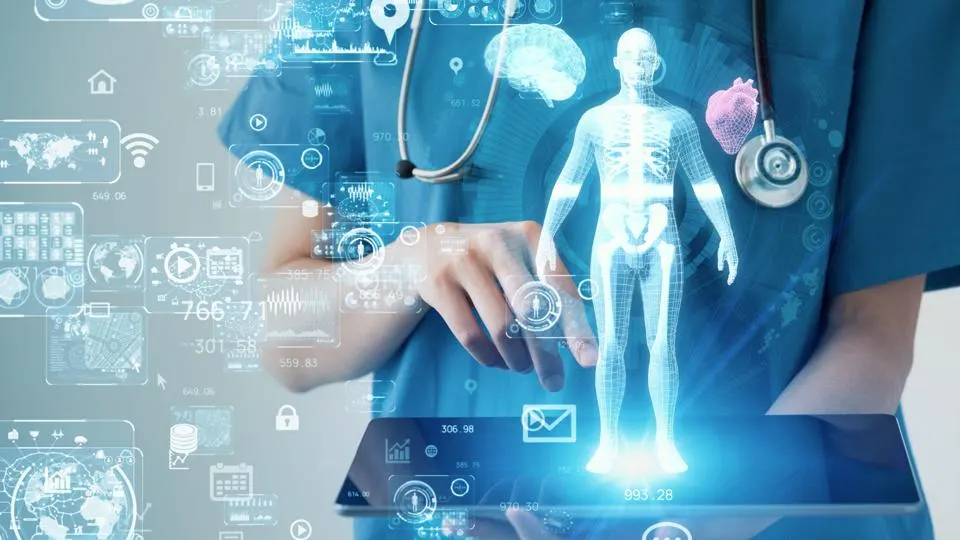
At the forefront of this healthcare revolution is Artificial Intelligence (AI), a technological force with the potential to reshape the entire patient care paradigm. AI, often associated with futuristic concepts, is not just a buzzword but a tangible solution to some of the most pressing issues faced by the healthcare sector. By leveraging machine learning algorithms, predictive analytics, and advanced data processing capabilities, AI is proving to be a catalyst for innovation across various facets of healthcare delivery.
Current Challenges in Healthcare
The healthcare industry is facing a multitude of challenges that disrupt the delivery of high-quality, accessible, and affordable care. These challenges are complex and multifaceted, and they often intersect with each other. Let’s delve into some of the most pressing challenges facing healthcare today:
Rising Healthcare Costs
The financial strain on healthcare systems globally has reached unprecedented levels, raising concerns about sustainability and accessibility. Escalating costs of medical services, pharmaceuticals, and administrative overheads contribute to a scenario where quality healthcare becomes a luxury for some rather than a universal right. The burden of these rising costs falls not only on patients but also on healthcare providers and payers, prompting a critical examination of existing financial models and the urgent need for cost-effective solutions.
Limited Access to Medical Services
While medical advancements have expanded the scope of available treatments, the unequal distribution of healthcare resources remains a significant challenge. Rural and underserved urban areas often face a scarcity of medical facilities, leading to delayed or inadequate healthcare access. Addressing this disparity requires innovative strategies, with technology, particularly telemedicine, emerging as a promising tool to bridge the gap and ensure that quality healthcare is accessible to all, regardless of geographical location.
Data Management and Interoperability Issues
The vast amount of health data generated daily holds immense potential for improving patient care, but the lack of standardized data management and interoperability hampers its effective use. Electronic Health Records (EHRs) exist in silos, hindering seamless information exchange between healthcare providers. This fragmentation not only compromises patient safety but also impedes the development of comprehensive, data-driven healthcare solutions. Overcoming these challenges necessitates a concerted effort to establish interoperable systems that prioritize data security and patient privacy.
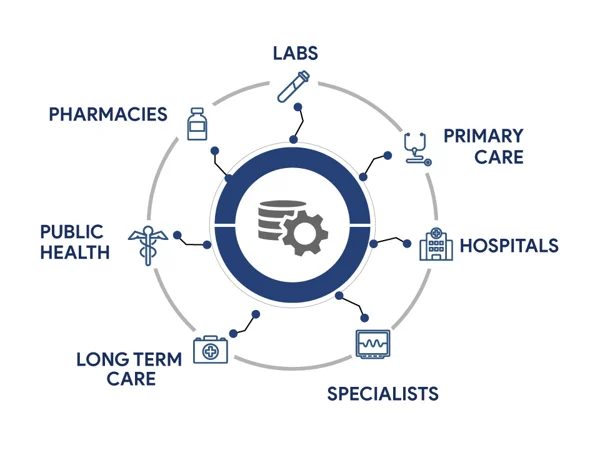
As we confront these pressing issues in healthcare, the integration of AI stands out as a promising avenue for solutions.
AI in Diagnostics and Early Detection
Medical imaging has long been a cornerstone of diagnostics, providing crucial insights into the human body’s intricacies. AI, with its capacity to analyze vast datasets swiftly, is revolutionizing medical imaging practices. Radiology, pathology, and even dermatology are witnessing a transformative shift as AI algorithms assist healthcare professionals in detecting anomalies, interpreting results, and making more accurate diagnoses. From identifying tumors in X-rays to pinpointing subtle changes in MRI scans, AI is augmenting the diagnostic capabilities of medical imaging, leading to earlier and more precise detection of diseases.
AI’s prowess in pattern recognition and data analysis is exemplified through a myriad of algorithms designed to detect diseases at their nascent stages. For instance, machine learning algorithms can analyze mammograms to identify potential signs of breast cancer, often achieving accuracy levels that rival or surpass human experts. In pathology, AI aids in the identification of abnormal cells, streamlining the diagnostic process and reducing the likelihood of oversight. These examples underscore the potential for AI to serve as a valuable ally in the quest for early disease detection, ultimately enhancing patient outcomes.
The integration of AI into diagnostics brings about a paradigm shift, significantly improving the accuracy and efficiency of medical assessments. By rapidly analyzing vast datasets, AI can identify subtle patterns and nuances that may escape the human eye. This not only expedites the diagnostic process but also minimizes the margin of error, leading to more reliable results. The synergy between AI and healthcare professionals results in a collaborative diagnostic approach, where the strengths of both humans and machines are harnessed to provide patients with more accurate diagnoses and timely interventions.
Personalized Treatment Plans
Personalized medicine, also known as precision medicine, is a rapidly evolving approach to healthcare that tailors treatment plans to the unique genetic, molecular, and lifestyle characteristics of each individual. AI plays a pivotal role in advancing personalized medicine by enabling the analysis of vast amounts of patient data and identifying patterns that inform personalized treatment decisions.
Traditional medical approaches often adopt a one-size-fits-all strategy, but every individual’s genetic makeup, lifestyle, and environmental factors contribute to a unique health profile. Personalized medicine acknowledges this diversity, aiming to tailor treatment plans to each patient’s specific characteristics. This approach not only enhances treatment efficacy but also minimizes adverse effects, fostering a more patient-centric healthcare model.
The ability of AI to process vast amounts of patient data quickly and discern intricate patterns empowers healthcare professionals to create highly individualized treatment plans. By analyzing genetic information, clinical records, and real-time health data, AI can identify optimal treatment options based on a patient’s unique biological markers. This data-driven approach ensures that treatments are not only effective but also consider the patient’s potential response and tolerance, ushering in a new era where medical interventions are tailored with unprecedented precision.
Telemedicine and Remote Patient Monitoring
Telemedicine and remote patient monitoring (RPM) have emerged as transformative tools in healthcare, revolutionizing the way patients receive care and enabling healthcare providers to extend their reach beyond traditional clinic settings. Telemedicine, facilitated by advancements in communication technology, transcends geographical barriers, bringing medical expertise to patients’ doorsteps. This is particularly crucial in addressing the challenges of limited access to healthcare services, especially in remote or underserved areas. Through virtual consultations, telemedicine not only enhances accessibility but also promotes early intervention and preventive care.
In the symbiotic relationship between AI and telemedicine, remote patient monitoring emerges as a critical enabler of proactive healthcare. AI-powered monitoring systems, coupled with wearable devices and sensors, provide continuous streams of real-time health data. This constant influx of information allows healthcare providers to remotely track patients’ vital signs, medication adherence, and overall well-being. By leveraging predictive analytics, AI can detect subtle deviations from baseline health parameters, enabling early intervention and reducing the likelihood of complications.
The adoption of virtual consultations not only improves access to healthcare but also develops a patient-centric approach. Patients can consult with healthcare professionals from the comfort of their homes, eliminating the need for travel and reducing the risk of exposure to infectious diseases. AI algorithms enhance the virtual healthcare experience by assisting in preliminary assessments, providing relevant medical information, and even supporting diagnostic processes. This collaborative approach ensures that the benefits of telemedicine extend beyond convenience to encompass quality and efficiency.
AI in Drug Discovery and Development
The conventional drug discovery process is full of challenges that impede the timely and cost-effective development of new medications. From the identification of potential drug candidates to rigorous testing and approval, the journey is lengthy and resource-intensive. High rates of attrition, where promising candidates fail in later stages of development, contribute to soaring research and development costs. The urgency to address pressing health needs demands a more efficient approach, and here is where AI steps in as a catalyst for innovation.
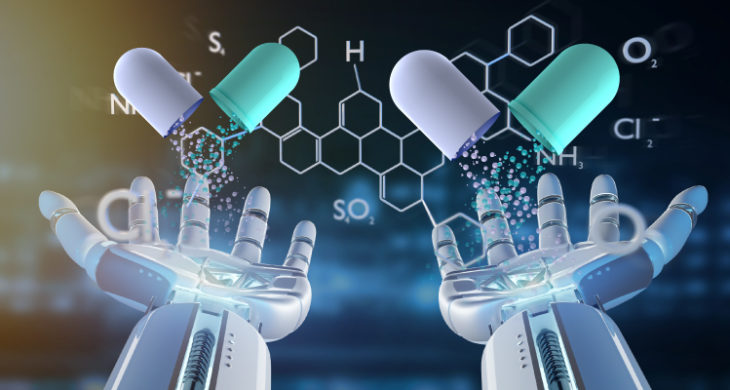
AI’s impact on drug discovery is revolutionary, offering solutions to long-standing challenges and expediting the identification of viable drug candidates. Machine learning algorithms analyze vast biological datasets, uncovering patterns and relationships that might elude traditional methods. Virtual screening, a process where AI predicts a compound’s potential based on its chemical structure, significantly streamlines the selection of candidates for laboratory testing. Moreover, AI enhances target identification, optimizes lead compounds, and facilitates predictive modeling, all contributing to a more agile and informed drug development pipeline.
Improving Operational Efficiency
The administrative burden within the healthcare system has long been a source of inefficiency, consuming valuable time and resources. AI interventions are reshaping the landscape by automating routine administrative tasks. From appointment scheduling to billing processes, AI-driven systems reduce the workload on healthcare staff, allowing them to focus on patient care. Natural Language Processing (NLP) algorithms enhance communication, facilitating smoother interactions between patients and administrative systems. The result is a more streamlined and responsive healthcare ecosystem that optimizes workflows and minimizes unnecessary delays.
Hospitals and healthcare facilities struggle with complex logistics and resource allocation challenges. AI steps in as a strategic partner, offering solutions to optimize workflows and resource management. Predictive analytics assist in forecasting patient admissions, enabling hospitals to allocate staff and resources more effectively. AI algorithms analyze historical data to identify patterns, ensuring that healthcare facilities are adequately prepared for fluctuations in patient numbers. This proactive approach not only enhances operational efficiency but also improves the overall quality of patient care.
The integration of AI into operational processes delivers tangible benefits in terms of cost-effectiveness and time savings. AI-driven systems can identify opportunities for cost reduction, whether through more efficient supply chain management or the optimization of treatment protocols. Additionally, automation of repetitive tasks translates to significant time savings for healthcare professionals, allowing them to dedicate more time to direct patient care. The cumulative effect is a healthcare system that operates more efficiently, delivering higher-quality care at a reduced cost.
Challenges and Limitations of AI in Healthcare
Despite the immense potential of AI to revolutionize healthcare, it is crucial to acknowledge the challenges and limitations associated with its implementation. Addressing these hurdles is essential for ensuring the responsible and effective integration of AI into clinical practice.
1. Potential for Bias
AI algorithms can perpetuate and amplify existing biases in healthcare, leading to unfair or discriminatory outcomes. Bias can arise from the data used to train AI models, which may reflect societal prejudices or lack of representation of certain patient populations.
- Algorithmic bias: AI algorithms may inherit biases from the data they are trained on, leading to biased predictions or decisions.
- Data bias: Biased data sources can lead to biased AI models, perpetuating existing disparities in healthcare outcomes.
- Lack of representation: Underrepresentation of certain patient groups in training data can lead to AI models that are less accurate or effective for those populations.
2. Concerns Regarding Reliability and Explainability
The complexity of AI models can raise concerns about their reliability and explainability. While AI algorithms can produce impressive results, understanding the reasoning behind their decisions can be challenging.
- Black box nature: The complex internal workings of AI models can make it difficult to understand how they arrive at their conclusions, hindering their trustworthiness and interpretability.
- Lack of transparency: The lack of transparency regarding AI model development and decision-making processes can raise concerns about accountability and potential errors.
- Explainability challenges: Explaining AI-driven predictions or decisions can be challenging, especially for complex models, making it difficult for healthcare providers to fully understand and trust the results.
3. Ethical Considerations and Data Privacy
The use of AI in healthcare raises significant ethical considerations and concerns about data privacy and patient confidentiality.
- Data privacy and security: The collection, storage, and use of vast amounts of patient data raise concerns about data privacy and security, requiring robust safeguards to protect sensitive information.
- Informed consent and patient autonomy: Obtaining informed consent from patients for AI-driven data collection and analysis is crucial to respect patient autonomy and ensure transparency.
- Fairness and non-discrimination: The implementation of AI in healthcare must be guided by principles of fairness and non-discrimination, ensuring that AI does not exacerbate existing disparities or introduce new forms of bias.
4. Continuous Refinement and Improvement of AI Systems
AI systems are not static; they require continuous refinement and improvement to maintain their effectiveness and reliability.
- Ongoing development and validation: AI models must be continuously updated and validated with new data to ensure their accuracy and generalizability across diverse patient populations.
- Human oversight and intervention: AI should be used as a tool to augment human expertise, not as a replacement for clinical judgment. Human oversight is crucial to ensure AI-driven decisions are consistent with clinical guidelines and patient values.
- Adaptability to the changing healthcare landscape: AI systems should be adaptable to the evolving healthcare landscape, incorporating new discoveries, treatment modalities, and clinical guidelines.
- Addressing potential pitfalls: Recognizing and addressing potential pitfalls, such as overfitting, data dependency, and lack of interpretability, is essential for the responsible development and implementation of AI in healthcare.
Future Prospects and Innovations
The future of AI in healthcare holds immense promise for transformative advancements in patient care, with several emerging trends shaping the future of the industry. NLP is becoming increasingly sophisticated, enabling more nuanced interactions between AI systems and healthcare professionals. Virtual health assistants, powered by AI, are expected to play a more significant role in patient engagement and support. Moreover, the integration of AI with other emerging technologies, such as augmented reality and virtual reality, holds the potential to enhance medical training, diagnosis, and treatment planning.
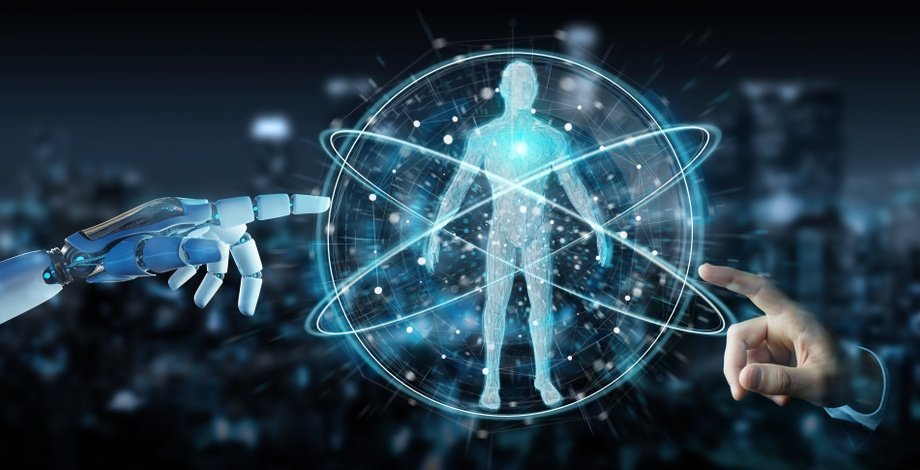
As we look ahead, envisioning a future where AI redefines patient care involves a holistic integration of technology and human expertise. Personalized medicine, facilitated by AI’s analytical power, is expected to become more commonplace, tailoring treatments to individual genetic profiles and lifestyle factors. AI-driven predictive analytics will likely play a crucial role in preventing diseases by identifying at-risk individuals and enabling proactive interventions. The collaborative partnership between healthcare professionals and AI is poised to create a healthcare ecosystem that is not only more efficient but also more compassionate and patient-centered.
Conclusion
The transformative impact of AI on the future of healthcare is profound. From enhancing diagnostics and personalizing treatment plans to improving operational efficiency, the integration of artificial intelligence is reshaping the healthcare landscape. Virtual consultations and remote patient monitoring bring healthcare to new frontiers, transcending geographical barriers and increasing accessibility.
While we celebrate the strides made, it’s essential to recognize that the journey is ongoing. The challenges of bias, ethical considerations, and the need for continuous refinement underscore the importance of ongoing research and collaboration. As AI in healthcare evolves, interdisciplinary efforts between technologists, healthcare professionals, ethicists, and policymakers will be pivotal in steering the trajectory of this transformative wave.
OphyCare is one of the digital health infrastructure companies that has been making significant developments in the realm of AI-powered healthcare. Their digital platform allows healthcare professionals to record, manage, and analyze data efficiently with minimum manual effort. Moreover, Sadiq – an AI-powered chatbot – is one of the most important components of this ecosystem. This smartly designed bot acts as a diagnosis mapping assistant that enables second-tier workers to detect disease at early stages.
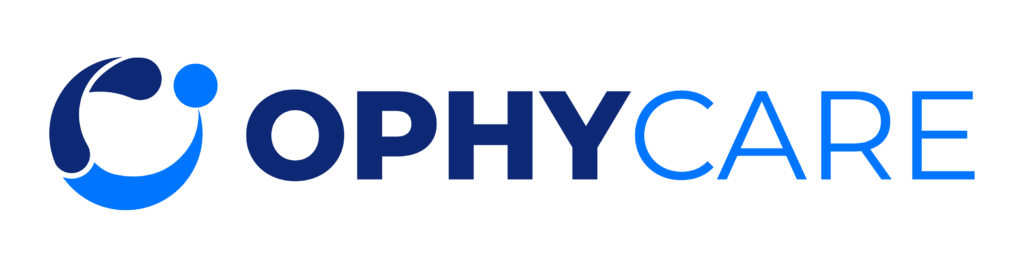